主讲人简介:
祝烨,澳大利亚迪肯大学信息技术学院计算机科学高级讲师(等同北美副教授),博士生导师。2017年于澳大利亚蒙纳士大学获得人工智能博士学位并取得全校年度最佳毕业论文Mollie Holman Medal奖,之后在澳大利亚迪肯大学从事数据挖掘相关研究与教学工作至今。他是IEEE高级会员和ACM会员。他的研究工作主要集中在聚类分析、异常检测、相似性学习及其在模式识别中的应用。祝博士在计算机顶级会议和期刊上发表了50多篇论文,包括SIGKDD、IJCAI、VLDB、AAAI、ICDM、TKDE、AIJ、ISJ、PRJ、JAIR和MLJ等。他担任许多知名国际会议的程序委员会主席和程序委员,如SIGKDD、AAAI、IJCAI和 PAKDD等。此外,他还获得了多个国家级跨学科和工业大型项目的资助,近期在迪肯大学荣获杰出青年研究员奖和优秀教学奖。
讲座(一)
讲座时间:10月23日9:00-11:30
讲座地点:管楼G216
讲座题目:Revolutionizing Anomaly Detection: Approaches and Guidelines
讲座摘要:
Anomaly detection plays a central role in data mining and remains a prominent subject of research in various areas of artificial intelligence. Its importance is underscored by its wide range of applications, including the identification of anomalous climatic events, mechanical malfunctions, potential threats, fraudulent activities, and malicious URLs. Dr Ye Zhu's presentation will provide a comprehensive overview of anomaly detection techniques, covering both shallow and deep learning-based methods, accompanied by in-depth explanations. Dr Zhu will explain the basic principles, objectives, underlying assumptions, and advantages and disadvantages of state-of-the-art anomaly detection approaches. He will also present several systematic approaches aimed at providing explanations for anomalies detected by deep learning models. In addition, Dr Zhu will explore the connections between conventional shallow methods and innovative deep techniques, and provide practical guidance on how to select the most appropriate outlier detection method for different scenarios.
讲座(二)
讲座时间:10月23日14:00-16:30
讲座地点:管楼G216
讲座题目:Clustering massive datasets for pattern recognition
讲座摘要:
Clustering is a powerful technique for discovering patterns and hidden structures in data. However, many real-world datasets contain clusters with varied densities and irregular shapes, which pose challenges for traditional clustering algorithms. Kernel-based clustering is a technique designed to identify non-linearly separable clusters with irregular shapes, but they have high computational costs. In this talk, I will present some recent advances in kernel-based clustering that use an adaptive distributional kernel to overcome the limitations of existing clustering algorithms. These methods can achieve better clustering quality with lower computational cost than existing kernel-based algorithms and can handle large datasets efficiently. Their applications can range from image and speech processing to gene pattern extraction, community detection and market segmentation.
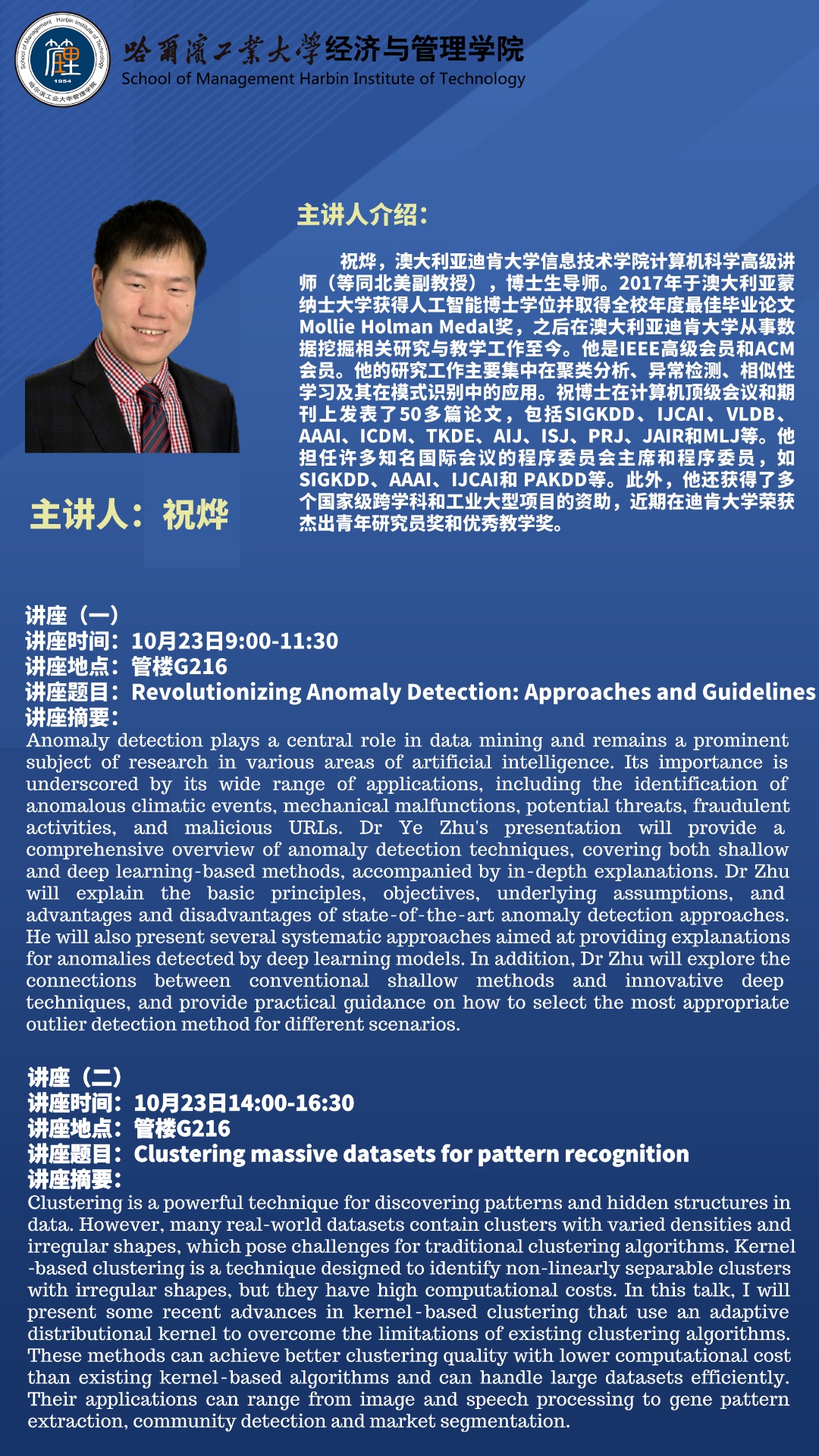