讲座时间:2023年9月8日(星期五),上午9:00-9:50
讲座地点:科学园2H 216
讲座题目:Deep Approaches to Tourism Demand Forecasting
报告人简介:
Prof. Gang Li is a full professor and university academic board member at Deakin University, Australia. His research includes data privacy, robust machine learning, group behavior analysis, and business intelligence. He holds international patents, and his research has been funded by various agencies, including ARC, India DST Sparc Scheme and Hong Kong GRF. Prof. Li serves as the chair for IEEE Task Force on Educational Data Mining (2020-2023), and on the IEEE Data Mining and Big Data Analytics Technical Committee (2017-2019 Vice Chair), IEEE Enterprise Information Systems Technical Committee. He is experienced as the general chair and program-committee co-chairs role for many CCF and CORE recommended conferences, including KSEM (2017, 2018), ISCC (2021), and HSPR (2023) etc. He is on the editorial board of prestigious journals including Decision Support Systems, Tourism Management, Journal of Travel Research, Asia Pacific Journal of Tourism Research.
讲座摘要:
As tourism researchers continue to search for solutions to determine the best possible forecasting performance, it is important to understand the maximum predictivity achieved by models, as well as how various data characteristics influence the maximum predictivity. Drawing on information theory, the predictivity of tourism demand data is quantitatively evaluated and beneficial for improving the performance of tourism demand forecasting. The methods are available for univariate and multi-variate data.
Then we will present a series of deep approaches to tourism demand forecasting - deep learning models, decomposition method, and group pooling method. The empirical results demonstrated that the deep learning approach significantly outperforms alternative models. Moreover, the construction and identification of highly relevant features from the proposed deep network architecture provide practitioners with a means of understanding the relationships between various tourist demand forecasting factors and tourist arrival volumes.
欢迎感兴趣的老师和同学参加!
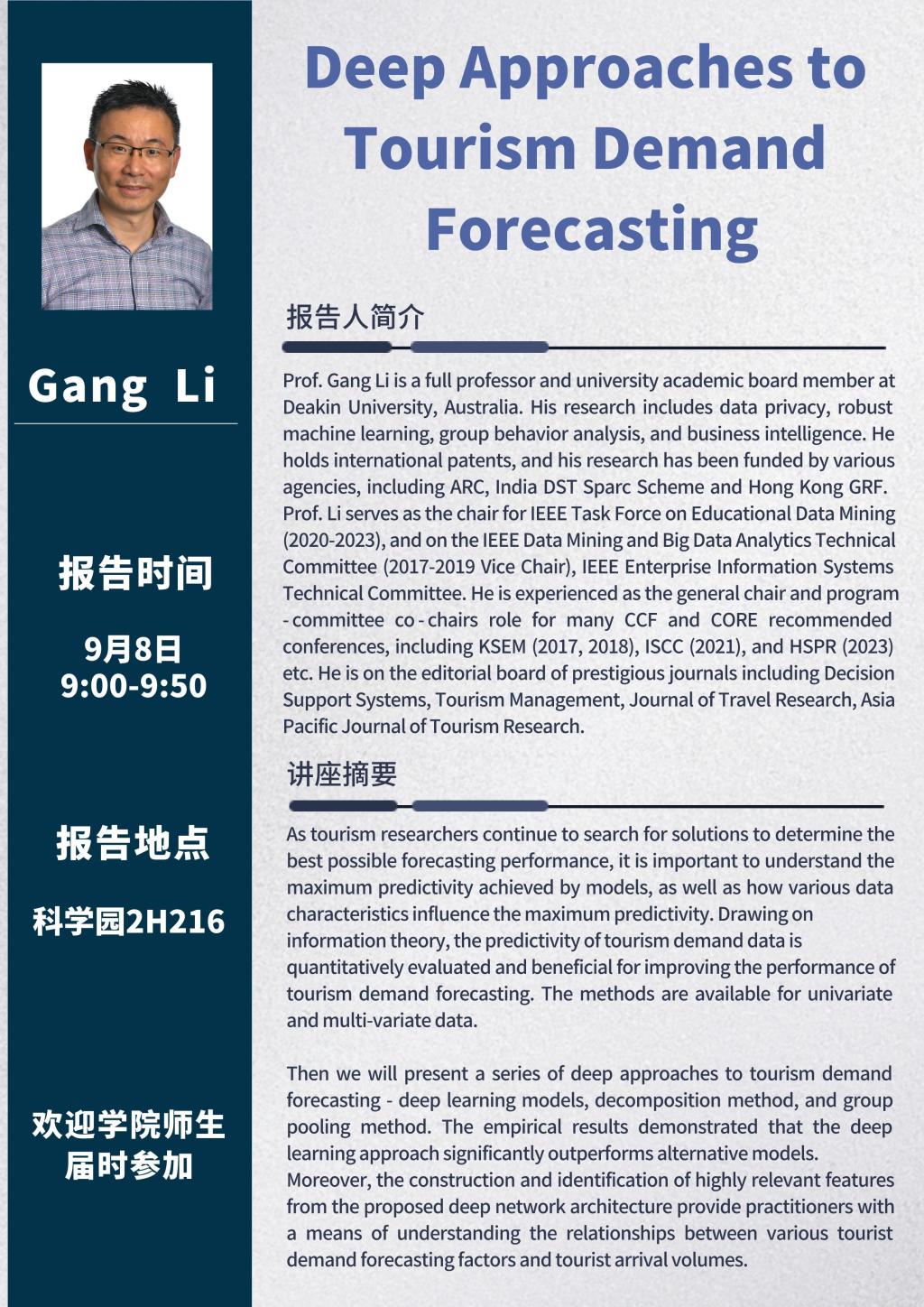